Nasem Badreldin
Dr. Nasem Badreldin

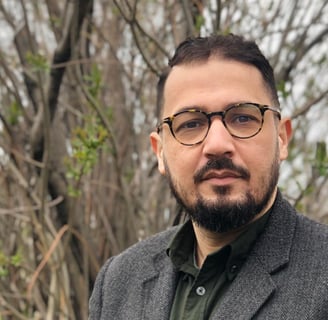
Nasem Badreldin is an assistant professor in digital agronomy at the University of Manitoba, Canada. He received his PhD in Geography from Ghent University (UGent) in Belgium (December 2013) and his MSc in Physical Land Resources from the Faculty of Bioscience Engineering in UGent (September 2008). He worked previously as a Postdoctoral Fellow at the Center for Earth Observation Sciences (CEOS) in the Dept. of Earth and Atmospheric Sciences at the University of Alberta in Canada and at the Dept. of Soil Science at the University of Manitoba and the University of Guelph in Canada, and as a Modeling Analyst in the Cumulative Impacts and Science Branch at the Saskatchewan Ministry of Environment.
Education
• PhD (Geography), Ghent University, Gent Belgium
• MSc. (Soil Science), Ghent University, Gent Belgium
• BSc (Environmental Agricultural Sciences), Suez Canal University, North Sinai, Egypt
Areas of Interest
• Digital soil mapping
• Modelling spatiotemporal landscape dynamics
• Monitoring crop growth and yield using various remote sensing technology
• Artificial intelligence applications in crop production and protection
• Precision farming development for site-specific management
• Digital soil mapping
• Modelling spatiotemporal landscape dynamics
• Monitoring crop growth and yield using various remote sensing technology
• Artificial intelligence applications in crop production and protection
• Precision farming development for site-specific management
Latest Publications
• Estimating the spatial distribution of soil volumetric water content in an agricultural field employing remote sensing and other auxiliary data under different tillage management practices. Soil Use and Management, 40, e12981. https://doi.org/10.1111/sum.12981
• Assessment of remotely sensed inventories for land cover classification of public grasslands in Manitoba, Canada. Grass and Forage Science, 78(4), 590–601. https://doi.org/10.1111/gfs.12631
• Integrating Active and Passive Remote Sensing Data for Mapping Soil Salinity Using Machine Learning and Feature Selection Approaches in Arid Regions. Remote Sensing. 2023, 15, 1751. https://doi.org/10.3390/rs15071751
• Mapping Grasslands in Mixed Grassland Ecoregion of Saskatchewan Using Big Remote Sensing Data and Machine Learning. Remote Sensing. 2021; 13(24):4972. https://doi.org/10.3390/rs13244972
تواصل معنا
العنوان:
رقم 10، بلوك 28037، المقاطعة الثالثة، مدينة العبور، مصر.